This recent interview with Gabe Newell of Valve caught our interest because it’s so rare that a game developer talks publicly about the potential of physiological computing to enhance the experience of gamers. The idea of using live physiological data feeds in order to adapt computer games and enhance game play was first floated by Kiel in these papers way back in 2003 and 2005. Like Kiel, in my writings on this topic (Fairclough, 2007; 2008 – see publications here), I focused exclusively on two problems: (1) how to represent the state of the player, and (2) what could the software do with this representation of the player state. In other words, how can live physiological monitoring of the player state inform real-time software adaptation? For example, to make the game harder or to increase the music or to offer help (a set of strategies that Kiel summarised in three categories, challenge me/assist me/emote me)- but to make these adjustments in real time in order to enhance game play.
physiological computing
Functional vocabulary: an issue for Emotiv and Brain-Computer Interfaces
The Emotiv system is a EEG headset designed for the development of brain-computer interfaces. It uses 12 dry electrodes (i.e. no gel necessary), communicates wirelessly with a PC and comes with a range of development software to create applications and interfaces. If you watch this 10min video from TEDGlobal, you get a good overview of how the system works.
First of all, I haven’t had any hands-on experience with the Emotiv headset and these observations are based upon what I’ve seen and read online. But the talk at TED prompted a number of technical questions that I’ve been unable to satisfy in absence of working directly with the system.
Continue reading
In the shadow of the polygraph
I was reading this short article in The Guardian today about the failure of polygraph technologies (including fMRI versions and voice analysis) to deliver data that was sufficiently robust to be admissible in court as evidence. Several points made in the article prompted a thought that the development of physiological computing technologies, to some extent, live in the shadow of the polygraph.
Think about it. Both the polygraph and physiological computing aim to transform personal and private experience into quantifiable data that may be observed and assessed. Both capture unconscious physiological changes that may signify hidden psychological motives and agendas, subconscious or otherwise – and of course, both involve the attachment of sensor apparatus. The convergence between both technologies dictates that both are notoriously difficult to validate (hence the problems of polygraph evidence in court) – and that seems true whether we’re talking about the use of the P300 for “brain fingerprinting” or the use of ECG and respiration to capture a specific category of emotion.
Whenever I do a presentation about physiological computing, I can almost sense antipathy to the concept from some members of audience because the first thing people think about is the polygraph and the second group of thoughts that logically follow are concerns about privacy, misuse and spying. To counter these fears, I do point out that physiological computing, whether it’s a game or a means of adapting a software agent or a brain-computer interface, has been developed for very different purposes; this technology is intended for personal use, it’s about control for the individual in the broadest sense, e.g. to control a cursor, to promote reflection and self-regulation, to make software reactive, personalised and smarter, to ensure that the data protection rights of the individual are preserved – especially if they wish to share their data with others.
But everyone knows that any signal that can be measured can be hacked, so even capturing these kinds of physiological data per se opens the door for spying and other profound invasions of privacy.
Which takes us inevitably back in the shadow of the polygraph.
I’m sure attitudes will change if the right piece of technology comes along that demonstrates the up side of physiological computing. But if early systems don’t take data privacy seriously, as in very seriously, the public could go cold on this concept before the systems have had a chance to prove themselves in the marketplace.
For musings on a similar theme, see my previous post Designing for the Guillable.
The Extended Nervous System
I’d like to begin the new year on a philosophical note. A lot of research in physiological computing is concerned with the practicalities of developing this technology. But what about the conceptual implications of using these systems (assuming that they are constructed and reach the marketplace)? At a fundamental level, physiological computing represents an extension of the human nervous system. This is nothing new. Our history is littered with tools and artifacts, from the plough to the internet, designed to extend the ‘reach’ of human senses capabilities. As our technology becomes more compact, we become increasingly reliant on tools to augment our cognitive capacity. This can be as trivial as using the address book on a mobile phone as a shortcut to “remembering” a friend’s number or having an electronic reminder of an imminent appointment. This kind of “scaffolded thinking” (Clark, 2004) represents a merger between a human limitation (long-term memory) and a technological solution, we’ve effectively subcontracted part of our internal cognitive store to an external silicon one. Andy Clark argues persuasively in his book that these human-machine mergers are perfectly natural consequence of human-technology co-evolution.
If we use technology to extend the human nervous system, does this also represent a natural consequence of the evolutionary trajectory that we share with machines? It is one thing to delegate information storage to a machine but granting access to the central nervous system, including the inner sanctum of the brain, represents a much more intimate category of human-machine merger.
In the case of muscle interfaces, where EMG activity or eye movements function as proxies of a mouse or touchpad input, I feel the nervous system has been extended in a modest way – gestures are simply recorded at a different place, rather than looking and pointing, you can now just look. BCIs represent a more interesting case. Many are designed to completely circumvent the conventional motor component of input control. This makes BCIs brilliant candidates for assistive technology and effective usage of a BCI device feels slightly magical – because it is the ultimate in remote control. But like muscle interfaces, all we have done is create an alternative route for human-computer input. The exciting subtext to BCI use is how the user learns to self-regulate brain activity in order to successfully operate this category of technology. The volitional control of brain activity seems like an extension of the human nervous system in my view (or to be more specific, an extension of how we control the human nervous system), albeit one that occurs as a side effect or consequence of technology use.
Technologies based on biofeedback mechanics, such as biocybernetic adaptation and ambulatory monitoring, literally extend the human nervous system by transforming a feeling/thought/experience that is private, vague and subjective into an observable representation that is public, quantified and objective. In addition, biocybernetic systems that monitor changes in physiology to trigger adaptive system responses take the concept further – these systems don’t merely represent the activity of the nervous system, they are capable of acting on the basis of this activity, completely bypassing human awareness if necessary. That prospect may alarm many but one shouldn’t be too disturbed – the autonomic nervous system routinely does hundreds of things every minute just to keep us conscious and alert – without ever asking or intruding on consciousness. Of course the process of autonomic control can run amiss, take panic attacks as one example, and it is telling that biofeedback represents one way to correct this instance of autonomic malfunction. The therapy works by making a hidden activity quantifiable and open to inspection, and in doing so, provides the means for the individual to “retrain” their own autonomic system via conscious control. This dynamic runs through those systems concerned with biocybernetic control and ambulatory monitoring. Changes at the user interface provide feedback on emotion or cognition and invite the user to extend self-awareness, and in doing so, to enhance control over their own central nervous systems. As N. Katherine Hayles puts it in her book on posthumanism: “When the body is integrated into a cybernetic circuit, modification of the circuit will necessarily modify consciousness as well. Connected to multiple feedback loops to the objects it designs, the mind is also an object of design.”
So, really what we’re talking about is extending our human nervous systems via technology and in doing so, enhancing our ability to self-regulate our human nervous systems. To slightly adapt a phrase from the autopoietic analysis of the nervous system, we are observing systems observing ourselves observing (ourselves).
It has been argued by Rosalind Picard among others that increased self-awareness and self-control of bodily states is a positive aspect of this kind of technology. In some cases, such as anger management and stress reduction, I can see clear arguments to support this position. On the other hand, I can also see potential for confusion and distress due to disembodiment (I don’t feel angry but the computer says I do – so which is me?) and invasion of privacy (I know you say you’re not angry but the computer says you are).
If we are to extend the nervous system, I believe we must also extend our conception of the self – beyond the boundaries of the skull and the skin – in order to incorporate feedback from a computer system into our strategies for self-regulation. But we should not be sucked into a simplistic conflicts by these devices. As N. Katherine Hayles points out, border crossings between humans and machines are achieved by analogy, not simple re-representation – the quantified self out there and the subjective self in here occupy different but overlapping spheres of experience. We must bear this in mind if we, as users of this technology, are to reconcile the plentitude of embodiment with the relative sparseness of biofeedback.
Categories of Physiological Computing
In my last post I articulated a concern about how the name adopted by this field may drive the research in one direction or another. I’ve adopted the Physiological Computing (PC) label because it covers the widest range of possible systems. Whilst the PC label is broad, generic and probably vague, it does cover a lot of different possibilities without getting into the tortured semantics of categories, sub-categories and sub- sub-categories.
I’ve defined PC as a computer system that uses real-time bio-electrical activity as input data. At one level, moving a mouse (or a Wii) with your hand represents a form of physiological computing as do physical interfaces based on gestures – as both are ultimately based on muscle potentials. But that seems a little pedantic. In my view, the PC concept begins with Muscle Interfaces (e.g. eye movements) where the electrical activity of muscles is translated into gestures or movements in 2D space. Brain-Computer Interfaces (BCI) represent a second category where the electrical activity of the cortex is converted into input control. Biofeedback represents the ‘parent’ of this category of technology and was ultimately developed as a control device, to train the user how to manipulate the autonomic nervous system. By contrast, systems involving biocybernetic adaptation passively monitor spontaneous activity from the central nervous system and translate these signals into real-time software adaptation – most forms of affective computing fall into this category. Finally, we have the ‘black box’ category of ambulatory recording where physiological data are continuously recorded and reviewed at some later point in time by the user or medical personnel.
I’ve tried to capture these different categories in the diagram below. The differences between each grouping lie on a continuum from overt observable physical activity to covert changes in psychophysiology. Some are intended to function as explicit forms of intentional communication with continuous feedback, others are implicit with little intentionality on the part of the user. Also, there is huge overlap between the five different categories of PC: most involve a component of biofeedback and all will eventually rely on ambulatory monitoring in order to function. What I’ve tried to do is sketch out the territory in the most inclusive way possible. This inclusive scheme also makes hybrid systems easier to imagine, e.g. BCI + biocybernetic adaptation, muscle interface + BCI – basically we have systems (2) and (3) designed as input control, either of which may be combined with (5) because it operates in a different way and at a different level of the HCI.
As usual, all comments welcome.
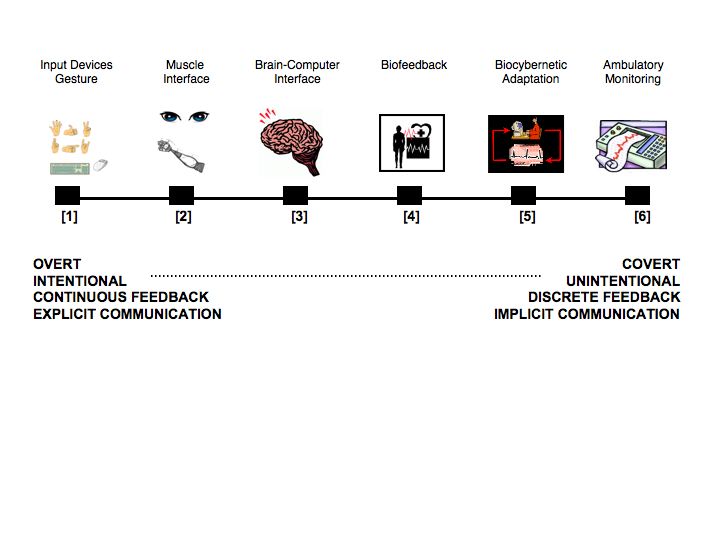
Five Categories of Physiological Computing
What’s in a name?
I attended a workshop earlier this year entitled aBCI (affective Brain Computer Interfaces) as part of the ACII conference in Amsterdam. In the evening we discussed what we should call this area of research on systems that use real-time psychophysiology as an input to a computing system. I’ve always called it ‘Physiological Computing’ but some thought this label was too vague and generic (which is a fair criticism). Others were in favour of something that involved BCI in the title – such as Thorsten Zander‘s definitions of passive vs. active BCI.
As the debate went on, it seemed that we were discussing was an exercise in ‘branding’ as opposed to literal definition. There’s nothing wrong with that, it’s important that nascent areas of investigation represent themselves in a way that is attractive to potential sponsors. However, I have three main objections to the BCI label as an umbrella term for this research: (1) BCI research is identified with EEG measures, (2) BCI remains a highly specialised domain with the vast majority of research conducted on clinical groups and (3) BCI is associated with the use of psychophysiology as a substitute for input control devices. In other words, BCI isn’t sufficiently generic to cope with: autonomic measures, real-time adaptation, muscle interfaces, health monitoring etc.
My favoured term is vague and generic, but it is very inclusive. In my opinion, the primary obstacle facing the development of these systems is the fractured nature of the research area. Research on these systems is multidisciplinary, involving computer science, psychology and engineering. A number of different system concepts are out there, such as BCI vs. concepts from affective computing. Some are intended to function as alternative forms of input control, others are designed to detect discrete psychological states. Others use autonomic variables as opposed to EEG measures, some try to combine psychophysiology with overt changes in behaviour. This diversity makes the area fun to work in but also makes it difficult to pin down. At this early stage, there’s an awful lot going on and I think we need a generic label to both fully exploit synergies, and most importantly, to make sure nothing gets ruled out.
Overt vs. Covert Expression
This article in New Scientist on Project Natal got me thinking about the pros and cons of monitoring overt expression via sophisticated cameras and covert expression of psychological states via psychophysiology. The great thing about the depth-sensing cameras (summarised nicely by one commentator in the article as like having a Wii attached to each foot, hand and your hand) is that: (1) it’s wireless technology, (2) interactions are naturalistic, and (3) it’s potentially robust (provided nobody else walks into the camera view). Also, because it captures overt expression of body position/posture or changes in facial expression/voice tone (the second being muted as a phase two development), it measuring those signs and signals that people are usually happy to share their fellow humans – so the feel of the interaction should be as naturalistic as a regular discourse.
So why bother monitoring psychophysiology in real time to represent the user? Let’s face it – there are big question marks over its reliability, it’s largely unproven in the field and normally involves attaching wires to the person – even if they are wearable.
But to view a face-off between the two approaches in terms of sensor technology is missing the point. The purpose of depth cameras is to give computer technology a set of eyes and ears to perceive & respond to overt visual or vocal cues from the user. Whilst psychophysiological methods have been developed to capture covert changes that remain invisible to the eye. For example, a camera system may detect a frown in response to an annoying email whereas a facial EMG recording will often detect increased activity from the corrugator or frontalis (i.e. the frown muscles) regardless of any change on the person’s face.
One approach is geared up to the detection of visible cues whereas the physiological computing approach is concerned with invisible changes in brain activity, muscle tension and autonomic activity. That last sentence makes the physiological approach sound superior, doesn’t it? But the truth is that both approaches do different things, and the question of which one is best depends largely on what kind of system you’re trying to build. For example, if I’m building an application to detect high levels of frustration in response to shoot-em-up gameplay, perhaps overt behavioural cues (facial expression, vocal changes, postural changes) will detect that extreme state. On the other hand, if my system needed to resolve low vs. medium vs. high vs. critical levels of frustration, I’d have more confidence in psychophysiological measures to provide the necessary level of fidelity.
Of course both approaches aren’t mutually exclusive and it’s easy to imagine naturalistic input control going hand-in-hand with real-time system adaptation based on psychophysiological measures.
But that’s the next step – Project Natal and similar systems will allow us to interact using naturalistic gestures, and to an extent, to construct a representation of user state based on overt behavioural cues. In hindsight, it’s logical (sort of) that we begin on this road by extending the awareness of a computer system in a way that mimics our own perceptual apparatus. If we supplement that technology by granting the system access to subtle, covert changes in physiology, who knows what technical possibilities will open up?
Neurofeedback in Education
FutureLab have published a discussion paper entitled “Neurofeedback: is there a potential for use in education?” It’s interesting to read a report devoted to the practical uses of neurofeedback for non-clinical populations. In short, the report covers definitions of neurofeedback & example systems (including EEG-based games like Mindball and MindFlex) as background. Then, three potential uses of neurofeedback are considered: training for sports performance, training for artistic performance and training to treat ADHD. The report doesn’t draw any firm conclusions as might be expected given the absence of systematic research programmes (in education). Aside from flagging up a number of issues (intrusion, reliability, expense), it’s obvious that we don’t know how these techniques are best employed in an educational environment, i.e. how long do students need to use them? What kind of EEG changes are important? How might neurofeedback be combined with other training techniques?
As I see it, there are a number of distinct application domains to be considered: (1) neurofeedback to shift into the desired psychological state prior to learning experience or examination (drawn from sports neurofeedback), (2) adapting educational software in real-time to keep the learner motivated (to avoid disengagement or boredom), and (3) to teach children about biological systems using biofeedback games (self-regulation exercises plus human biology practical). I’m staying with non-clinical applications here but obviously the same approaches may be applied to ADHD.
(1) and (3) above both correspond to a traditional biofeedback paradigm where the user works with the processed biological signal to develop a degree of self-regulation, that hopefully with transfer with practice. (2) is more interesting in my opinion; in this case, the software is being adapted in order to personalise and optimise the learning process for that particular individual. In other words, an efficient psychological state for learning is being created in situ by dynamic software adaptation. This approach isn’t so good for encouraging self-regulatory strategies compared to traditional biofeedback, but I believe it is more potent for optimising the learning process itself.
Formalising the unformalisable
Research into affective computing has prompted a question from some in the HCI community about formalising the unformalisable. This is articulated in this 2005 paper by Kirsten Boehner and colleagues. In essence, the argument goes like this – given that emotion and cognition are embodied biopsychological phenomena, can we ever really “transmit” the experience to a computer? Secondly, if we try to convey emotions to a computer, don’t we just trivialise the experience by converting it into another type of cold, quantified information. Finally, hasn’t the computing community already had its fingers burned by attempts to have machines replicate cognitive phenomenon with very little results (e.g. AI research in the 80’s).
OK. The first argument seems spurious to me. Physiological computing or affective computing will never transmit an exact representation of private psychological events. That’s just setting the bar too high. What physiological computing can do is operationalise the psychological experience, i.e. to represent a psychological event or continuum in a quantified, objective fashion that should be meaningfully associated with the experience of that psychological event. As you can see, we’re getting into deep waters already here. The second argument is undeniable but I don’t understand why it is a criticism. Of course we are taking an experience that is private, personal and subjective and converting it into numbers. But that’s what the process of psychophysiological measurement is all about – moving from the realm of experience to the realm of quantified representation. After all, if you studied an ECG trace of a person in the midst of a panic attack, you wouldn’t expect to experience a panic attack yourself, would you? Besides, converting emotions into numbers is the only way a computer has to represent psychological status.
As for the last argument, I’m on unfamiliar ground here, but I hope the HCI community can learn from the past mistakes; specifically, being too literal and unrealistically ambitious. Unfortunately the affective computing debate sometimes seems to run down these well-trodden paths. I’ve read papers where researchers ponder how computers will ‘feel’ emotions or whether the whole notion of emotional computing is an oxymoron. Getting computers to represent the psychological status of users is a relative business that needs to take a couple of baby steps before we try and run.
Designing for the gullible
There’s a nice article in todays Guardian by Charles Arthur regarding user gullibility in the face of technological systems. In this case, he’s talking about the voice risk analysis (VRA) software used by local councils and insurance companies to detect fraud (see related article by same author), which performs fairly poorly when evaluated, but is reckoned by those bureaucrats who purchased the system to be a huge money-saver. The way it works is this – operator receives a probability that the claimant is lying (based on “brain traces in the voice” – in reality probably changes in the fundamental frequency and pitch of the voice), and on this basis, may elect to ask more detailed questions.
Charles Arthur makes the point that we’re naive and gullible when faced with a technological diagnosis. And this is fair point, whether it’s the voice analysis system or a physiological computing system providing feedback that you’re happy or tired or anxious. Why do we tend to yield to computerised diagnosis? In my view, you can blame science for that – in our positivist culture, cold objective numbers will always trump warm subjective introspection. The first experimental psychologist, Wilhem Wundt (1832-1920) pointed to this dichotomy when he distinguished between mediated and unmediated consciousness. The latter is linked to introspection whereas the former demands the intervention of an instrument or technology. If you go outside on an icy day and say to yourself “it’s cold today” – your consciousness is unmediated. If you supplement this insight by reading a thermometer “wow, two degrees below zero” – that’s mediated consciousness. One is broadly true from that person’s perspective whereas the other is precise from point of view of almost anyone.
The main point of today’s article is that we tend to trust technological diagnosis even when the scientific evidence supporting system performance is flawed (as is claimed in the case of the VRA system). Again, true enough – but in fairness, most users of the VRA didn’t get the chance to review the system evaluation data. The staff are trained to believe the system by the company rep who sold the system and trained them how to use it. From the perspective of the customers, insurance staff may have suddenly started to ask them a lot of detailed questions, which indicated their stories were not believed, which probably made the customers agitated and anxious, therefore raising the pitch of the voice and turning themselves from possibles to definites. The VRA system works very well in this context because nobody really knew how it worked or even whether it worked.
What does all this mean for physiological computing? First of all, system designers and users must accept that psychophysiological measurement will never give a perfect, isomorphic, one-to-one model of human experience. The system builds a model of the user state, not a perfect representation. Given this restriction, system designers must be clever in terms of providing feedback to the user. Explicit and continuous feedback from the system is likely to undermine the credibility of the system in the eyes of the user. Users of physiological computing systems must be sufficiently informed to understand that feedback from the system is an educated assessment.
The construction of physiological computing systems is a bridge-building exercise in some ways – a link between the nervous system and the computer chip. Unlike similar constructions, this bridge is unlikely to ever meet in the middle. For that to happen, the user must rely his or her gullibility to make the necessary leap of faith to close the circuit. Unrealistic expectation will lead to eventual disappointment and disillusionment, conservative cynicism and suspicious will leave the whole physiological computing concept stranded at the starting gate – it’s up to designers to build interfaces that lead the user down the middle path.