From the point of view of an outsider, the utility and value of computer technology that provides emotional feedback to the human operator is questionable. The basic argument normally goes like this: even if the technology works, do I really need a machine to tell me that I’m happy or angry or calm or anxious or excited? First of all, the feedback provided by this machine would be redundant, I already have a mind/body that keeps me fully appraised of my emotional status – thank you. Secondly, if I’m angry or frustrated, do you really think I would helped in any way by a machine that drew my attention to these negative emotions, actually that would be particularly annoying. Finally, sometimes I’m not quite sure how I’m feeling or how I feel about something; feedback from a machine that says you’re happy or angry would just muddy the waters and add further confusion.
affective computing
Physiological Computing: increased self-awareness or the fast track to a divided ego?
In last week’s excellent Bad Science article from The Guardian, Ben Goldacre puts his finger on a topic that I think is particularly relevant for physiological computing systems. He quotes press reports about MRI research into “hypoactive sexual desire response” – no, I hadn’t heard of it either, it’s a condition where the person has low libido. In this study women with the condition and ‘normals’ viewed erotic imagery in the scanner. A full article on the study from the Mail can be found here but what caught the attention of Bad Science is this interesting quote from one of the researchers involved: “Being able to identify physiological changes, to me provides significant evidence that it’s a true disorder as opposed to a societal construct.”
Valve experimenting with physiological input for games
This recent interview with Gabe Newell of Valve caught our interest because it’s so rare that a game developer talks publicly about the potential of physiological computing to enhance the experience of gamers. The idea of using live physiological data feeds in order to adapt computer games and enhance game play was first floated by Kiel in these papers way back in 2003 and 2005. Like Kiel, in my writings on this topic (Fairclough, 2007; 2008 – see publications here), I focused exclusively on two problems: (1) how to represent the state of the player, and (2) what could the software do with this representation of the player state. In other words, how can live physiological monitoring of the player state inform real-time software adaptation? For example, to make the game harder or to increase the music or to offer help (a set of strategies that Kiel summarised in three categories, challenge me/assist me/emote me)- but to make these adjustments in real time in order to enhance game play.
Better living through affective computing
I recently read a paper by Rosalind Picard entitled “emotion research for the people, by the people.” In this article, Prof. Picard has some fun contrasting engineering and psychological perspectives on the measurement of emotion. Perhaps I’m being defensive but she seemed to have more fun poking fun at the psychologists than the engineers, but the central impasse that she identified goes something like this: engineers develop sensor apparatus that can deliver a whole range of objective data whilst psychologists have decades of experience with theoretical concepts related to emotion, so why haven’t people really benefited from their union through the field of affective computing. Prof. Picard correctly identifies a reluctance on the part of the psychologists to define concepts with sufficient precision to aid the work of the engineers. What I felt was glossed over in the paper was the other side of the problem, namely the willingness of engineers to attach emotional labels to almost any piece of psychophysiological data, usually in the context of badly-designed experiments (apologies to any engineers reading this, but I wanted to add a little balance to the debate).
Continue reading
Categories of Physiological Computing
In my last post I articulated a concern about how the name adopted by this field may drive the research in one direction or another. I’ve adopted the Physiological Computing (PC) label because it covers the widest range of possible systems. Whilst the PC label is broad, generic and probably vague, it does cover a lot of different possibilities without getting into the tortured semantics of categories, sub-categories and sub- sub-categories.
I’ve defined PC as a computer system that uses real-time bio-electrical activity as input data. At one level, moving a mouse (or a Wii) with your hand represents a form of physiological computing as do physical interfaces based on gestures – as both are ultimately based on muscle potentials. But that seems a little pedantic. In my view, the PC concept begins with Muscle Interfaces (e.g. eye movements) where the electrical activity of muscles is translated into gestures or movements in 2D space. Brain-Computer Interfaces (BCI) represent a second category where the electrical activity of the cortex is converted into input control. Biofeedback represents the ‘parent’ of this category of technology and was ultimately developed as a control device, to train the user how to manipulate the autonomic nervous system. By contrast, systems involving biocybernetic adaptation passively monitor spontaneous activity from the central nervous system and translate these signals into real-time software adaptation – most forms of affective computing fall into this category. Finally, we have the ‘black box’ category of ambulatory recording where physiological data are continuously recorded and reviewed at some later point in time by the user or medical personnel.
I’ve tried to capture these different categories in the diagram below. The differences between each grouping lie on a continuum from overt observable physical activity to covert changes in psychophysiology. Some are intended to function as explicit forms of intentional communication with continuous feedback, others are implicit with little intentionality on the part of the user. Also, there is huge overlap between the five different categories of PC: most involve a component of biofeedback and all will eventually rely on ambulatory monitoring in order to function. What I’ve tried to do is sketch out the territory in the most inclusive way possible. This inclusive scheme also makes hybrid systems easier to imagine, e.g. BCI + biocybernetic adaptation, muscle interface + BCI – basically we have systems (2) and (3) designed as input control, either of which may be combined with (5) because it operates in a different way and at a different level of the HCI.
As usual, all comments welcome.
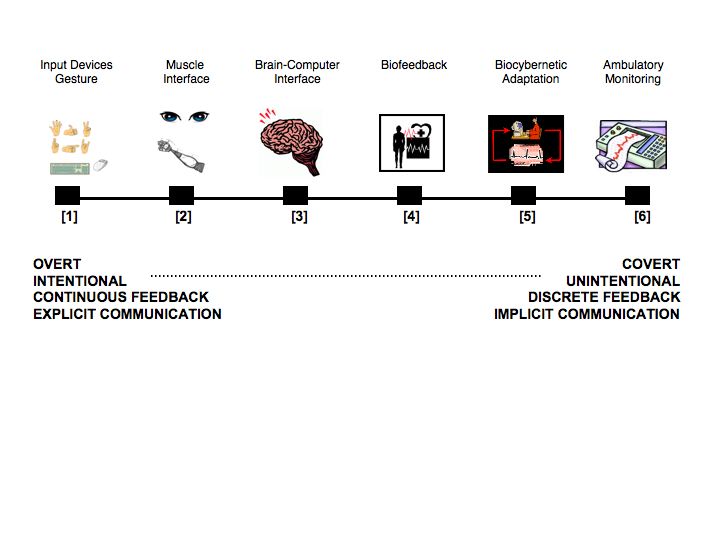
Five Categories of Physiological Computing
What’s in a name?
I attended a workshop earlier this year entitled aBCI (affective Brain Computer Interfaces) as part of the ACII conference in Amsterdam. In the evening we discussed what we should call this area of research on systems that use real-time psychophysiology as an input to a computing system. I’ve always called it ‘Physiological Computing’ but some thought this label was too vague and generic (which is a fair criticism). Others were in favour of something that involved BCI in the title – such as Thorsten Zander‘s definitions of passive vs. active BCI.
As the debate went on, it seemed that we were discussing was an exercise in ‘branding’ as opposed to literal definition. There’s nothing wrong with that, it’s important that nascent areas of investigation represent themselves in a way that is attractive to potential sponsors. However, I have three main objections to the BCI label as an umbrella term for this research: (1) BCI research is identified with EEG measures, (2) BCI remains a highly specialised domain with the vast majority of research conducted on clinical groups and (3) BCI is associated with the use of psychophysiology as a substitute for input control devices. In other words, BCI isn’t sufficiently generic to cope with: autonomic measures, real-time adaptation, muscle interfaces, health monitoring etc.
My favoured term is vague and generic, but it is very inclusive. In my opinion, the primary obstacle facing the development of these systems is the fractured nature of the research area. Research on these systems is multidisciplinary, involving computer science, psychology and engineering. A number of different system concepts are out there, such as BCI vs. concepts from affective computing. Some are intended to function as alternative forms of input control, others are designed to detect discrete psychological states. Others use autonomic variables as opposed to EEG measures, some try to combine psychophysiology with overt changes in behaviour. This diversity makes the area fun to work in but also makes it difficult to pin down. At this early stage, there’s an awful lot going on and I think we need a generic label to both fully exploit synergies, and most importantly, to make sure nothing gets ruled out.
Brain, Body and Bytes Workshop: CHI 2010
quantifying the self (again)
I just watched this cool presentation about blogging self-report data on mood/lifestyle and looking at the relationship with health. My interest in this topic is tied up in the concept of body-blogging (i.e. recording physiological data using ambulatory systems) – see earlier post. What’s nice about the idea of body-blogging is that it’s implicit and doesn’t require you to do anything extra, such as completing mood ratings or other self-reports. The fairly major downside to this approach comes in two varieties: (1) the technology to do it easily is still fairly expensive and associated software is cumbersome to use (not that it’s bad software, it’s just designed for medical or research purposes), and (2) continuous physiology generates a huge amount of data.
For the individual, this concept of self-tracking and self-quantifying is linked to increased self-awareness (to learn how your body is influenced by everyday events), and with self-awareness comes new strategies for self-regulation to minimise negative or harmful changes. My feeling is that there are certain times in your life (e.g. following a serious illness or medical procedure) when we have a strong motivation to quantify and monitor our physiological patterns. However, I see a risk of that strategy tipping a person over into hypochondria if they feel particularly vulnerable.
At the level of the group, it’s fascinating to see the seeds of a crowdsourcing idea in the above presentation. Therefore, people self-log over a period and share this information anonymously on the web. This activity creates a database that everyone can access and analyse, participants and researchers alike. I wonder if people would be as comfortable sharing heart rate or blood pressure data – provided it was submitted anonymously, I don’t see why not.
There’s enormous potential here for wearable physiological sensors to be combined with self-reported logging and both data sets to be combined online. Obviously there is a fidelity mismatch here; physiological data can be recorded in milliseconds whilst self-report data is recorded in hours. But some clever software could be constructed in order to aggregate the physiology and put both data-sets on the same time frame. The benefit of doing this for both researcher and participant is to explore the connections between (previously) unseen patterns of physiological responses and the experience of the individual/group/population.
For anyone who’s interested, here’s a link to another blog site containing a report from an event that focused on self-tracking technologies.
Audience Participation
A paper just published in IJHCS by Stevens et al (link to abstract) describes how members of the audience use a PDA to register their emotional responses in real-time during a number of dance performances. It’s an interesting approach to studying how emotional responses may converge and diverge during particular sections of a performance. The PDA displays a two-dimensional space with valence and activation representing emotion (i.e. Russell’s circumplex model). The participants were required to indicate their position within this space with a stylus at rate of two readings per second!
That sounds like a lot of work, so how about a physiological computing version where valence and activation are operationalised with real-time psychophysiology, e.g. a corrugator/zygomaticus reading for valence and blood pressure/GSR/heart rate for activation. Provided that the person remained fairly stationary, it could deliver the same kind of data with a higher level of fidelity and without the onerous requirement to do self-reports.
This system concept could really take off if you had 100s of audience members wired up for a theatre performance and live feedback of the ‘hive’ emotion represented on stage. This could be a backdrop projection or colour/intensity of stage lighting working as an en-masse biofeedback system. A clever installation could allow the performers to interact with the emotional representation of the audience – to check out the audience response or coerce certain responses.
Or perhaps this has already been done somewhere and I missed it.
Emotional HCI
Just read a very interesting and provocative paper entitled “How emotion is made and measured” by Kirsten Boehner and colleagues. The paper provides a counter-argument to the perspective that emotion should be measured/quantified/objectified in HCI and used as part of an input to an affective computing system or evaluation methodology. Instead they propose that emotion is a dynamic interaction that is socially constructed and culturally mediated. In other words, the experience of anger is not a score of 7 on a 10-point scale that is fixed in time, but an unfolding iterative process based upon beliefs, social norms, expectations etc.
This argument seems fine in theory (to me) but difficult in practice. I get the distinct impression the authors are addressing the way emotion may be captured as part of a HCI evaluation methodology. But they go on to question the empirical approach in affective computing. In this part of the paper, they choose their examples carefully. Specifically, they focus on the category of ‘mirroring’ (see earlier post) technology wherein representations of affective states are conveyed to other humans via technology. The really interesting idea here is that emotional categories are not given by a machine intelligence (e.g. happy vs. sad vs. angry) but generated via an interactive process. For example, friends and colleagues provide the semantic categories used to classify the emotional state of the person. Or literal representations of facial expression (a web-cam shot for instance) are provided alongside a text or email to give the receiver an emotional context that can be freely interpreted. This is a very interesting approach to how an affective computing system may provide feedback to the users. Furthermore, I think once affective computing systems are widely available, the interpretive element of the software may be adapted or adjusted via an interactive process of personalisation.
So, the system provides an affective diagnosis as a first step, which is refined and developed by the person – or even by others as time goes by. Much like the way Amazon makes a series of recommendations based on your buying patterns that you can edit and tweak (if you have the time).
My big problem with this paper was that a very interesting debate was framed in terms of either/or position. So, if you use psychophysiology to index emotion, you’re disregarding the experience of the individual by using objective conceptualisations of that state. If you use self-report scales to quantify emotion, you’re rationalising an unruly process by imposing a bespoke scheme of categorisation etc. The perspective of the paper reminded me of the tiresome debate in psychology between objective/quantitative data and subjective/qualitative data about which method delivers “the truth.” I say ‘tiresome’ because I tend towards the perspectivist view that both approaches provide ‘windows’ on a phenomenon, both of which have advantages and disadvantages.
But it’s an interesting and provocative paper that gave me plenty to chew over.